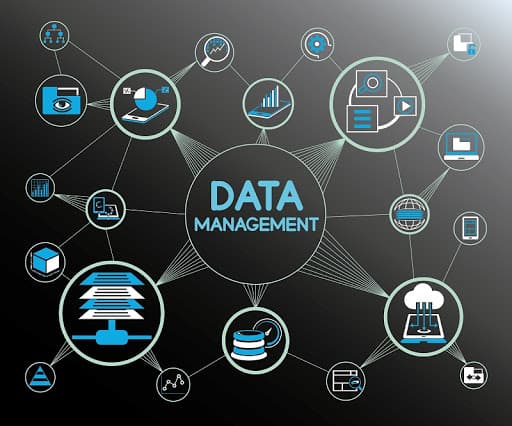
Professional data management is the key to success. The first step is when a company has decided to use data to control its marketing and sales activities and rely on automation. Because today’s modern technologies can only perform as well as the state of the database allows. Therefore, it is particularly important to ensure professional data management before the introduction of marketing automation. The planned automation processes can only be set up based on a complete 360 ° view of the customer. Professional data management increases efficiency and enables the customer to be offered an individualized and positive experience and thus increases a company’s competitiveness. Also, “gut decisions” can be measured, and scaling is possible more quickly. This blog shows what is meant by professional data management and provides ten valuable practical tips as a guide for successful implementation.
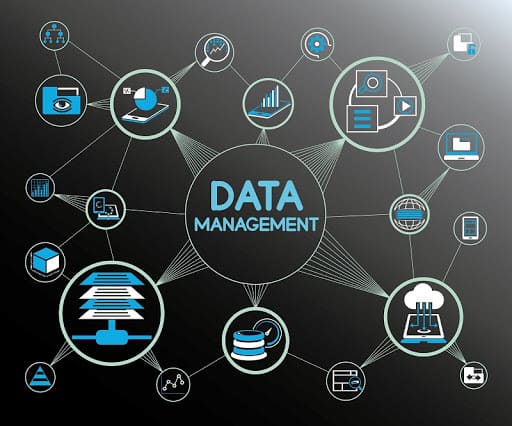
Data management – what is it?
The buzzword data management is a highly complex structure of technical measures linked to organizational processes and questions of the overall concept.
Data management supports companies in collecting data themselves and securely storing and making available data. Data is repeatedly lost in daily processes or gets stuck in silos. Organizational structures in which there are many highly specialized departments also favour the formation of silos. But technological barriers also ensure that not all parties involved have access to the data. This is the case, for example, when different departments use different software. Companies that use marketing automation are mainly dependent on good data management. Are all available customer data made available at a central point on the automation platform? This can better respond to the individual needs of each customer. Centrally stored, well-maintained customer profile data is the first step towards greater effectiveness of automated campaigns (see graphic).
Things to know about data
Data is not just information; it has a complex life cycle within a company and can be roughly divided into three categories:
- Behavioural data: e.g. social media and web interactions of (potential) customers
- Historical data: e.g. B. purchase history, support inquiries and all other interactions between customers and companies
- 360 ° view: all interactions between customers and companies are viewed across all channels
This data can, in turn, have four different states:
- The data used is kept up-to-date in the operational system.
- Analyzed data are no longer subject to changes but are only evaluated.
- Archived and deleted: data was saved in an archive or deleted from the system.
It is crucial for good data management to carefully define the rules that allow the data to pass from one phase of the life cycle to another. There is no one-size-fits-all solution here because every company has to define these phases based on its processes. When establishing these definitions, a distinction must also be made between the individual information objects’ criticality. After all, critical company information needs different treatment than non-critical information.
Get a grip on data management with these ten tips
Low data quality and poor data management are the biggest hurdles when generating ROI with marketing automation. The more data-driven marketing and sales are, the greater the importance of data management. Poorly maintained data has numerous disadvantages: it disrupts the user experience, makes sales less efficient, harms the credibility of a company’s marketing and ultimately causes business goals to be missed. The following ten tips should be understood as the first guide to help companies advance their data management.
Tip # 1: create a data overview
Companies obtain their data from various sources, or they already have data that is several years old. Companies that want to get into professional data management are then faced with a real “patchwork quilt” and must first provide basic orientation. To create a consistent database, companies should ask themselves the following three questions:
- “Do we use different formats or systems for the database?”
- «Where are our data stored? How safely and easily can we transfer this to other systems? “
- “Are the legal requirements met?”
Most companies face the challenge of having their data stored in different locations. The first thing to do is to create an overview of where the data comes from. All data sources from ERP or CRM to Excel sheets are collected for this purpose. Data silos that naturally develop in larger companies should also be included. These arise mainly when departments compete with each other and withhold information from each other. To avoid the formation of silos in the future, a rethink must occur so that the specific business goal comes to the fore again.
Tip # 2: centralize and cleanse data
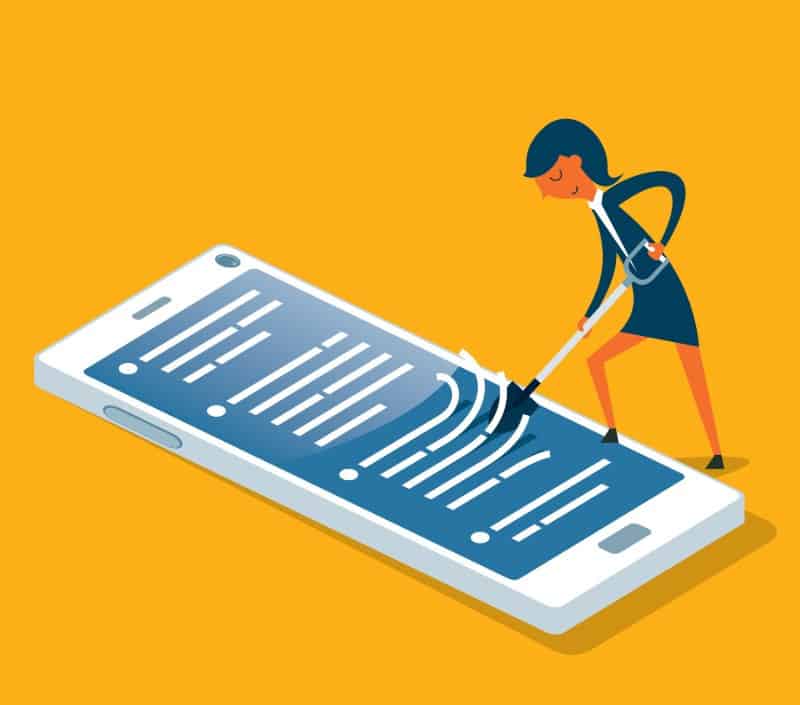
Once the data has been compiled, it is checked for quality, reliability and consistency. There are also special data quality tools that can automate this process, always considering the respective company’s requirements. Duplicates are removed, and – depending on the device – even missing data is added via the CRM. In this way, the data quality and the data relevance for the specific marketing needs can be maintained. In the course of data cleansing, it is determined which historical data are still relevant for storage. It is also important to clarify which of the existing data should be available for internal activities and be available for future customer communication. An inconsistent database, such as the wrong contact person, Includes incorrect information and incorrect content, reduces efficiency and increases wastage. Data acquisition and transmission are two additional sources of error. Intensive training of employees can remedy this.
Tip # 3: Selection of modern technology mix and integration with additional software
Good data management requires the appropriate tools to be integrated into the existing software infrastructure. Many marketing automation systems bring CRM functionalities and tools for data management and data analysis to make this step easier.
Therefore, when looking for a new CRM, an SME can often rely directly on a marketing automation system. This means that two methods do not have to be purchased, and license costs and interface projects can be saved.
It is worthwhile for a company to record the current system architecture and data flows to get an overview of the technological starting position. Based on this, it can be decided which systems should be replaced by modern technologies and which methods can be connected using an interface. This is how companies achieve the centralization of their data. But be careful: “Technology follows strategy”. This should not result in an IT project. Instead, a company should consider what it ultimately wants to achieve with this data and how sales and marketing activities are useful. Only when the strategy is in place is the technology mix put together in a tailor-made manner. For example, this is precisely the philosophy that we call Live 4results.
Tip # 4: analyze inventory data
Only after the data has been cleansed and updated can it be analyzed which customer groups predominate. Web examines help to get an idea of existing customers. For this purpose, data from analytics tools, but also their behaviour and conversions, are used. This is followed by a fundamental analysis that deals more with the customer than with his behaviour. The following questions play a role in such a more detailed customer analysis:
- How often do customers buy?
- The average number of orders per customer
- How many customers bought just once? If the customer only ordered once, did they have a bad experience? Didn’t you remember the shop? Did he go to the competitor? In all cases, there is some catching up to do.
- How many customers have bought more than three times? This group is a valuable asset from which much can be learned. If this group only makes up a small percentage, it should urgently take care of customer loyalty.
- New customers vs. Existing customers
Transaction data for data-based analysis
The data-based analysis is one of the essential analyzes and, above all, also includes inventory data. It examines sales, the average size of the shopping cart and, of course, what the customer has bought. Also, it gives valuable ideas for possible cross- and up-selling campaigns and shows which customer groups are in which size in the company. Customer surveys supplement this data, with which ultimately the buyer persona can be created for the company. In a further step, this helps to design appropriate measures and campaigns for the relevant groups. The analyzes all come from the transactional data, which is among the most reliable sources. The observation period should be at least one year. However, we do not recommend a period longer than three years, as both the customer and the product change over such a long period.
Tip # 5: segment the data
In the online world, companies often have no personal contact with their customers, so it is essential to create customer segments. Data is already sufficient to anticipate customer needs. They provide information about what customers are buying, how much they are spending, and what combination and time intervals they believe things. Segmentation ensures that an advertising campaign only reaches those target customers who can feel addressed. Effective segmentation provides that time and money flow to the right target group. If you know precisely what new customers, existing customers, and even inactive customers want, you can better meet their needs. Customer segmentation can be approached under various criteria. While some companies mainly use customer history to Sales funnel possible.
Behaviour-based segmentation is crucial.
Depending on the company, product or service, geographical segmentation criteria or behaviour can also be decisive. This is precisely a great advantage of marketing automation software. With the “behavior-based tracking” function, companies can see what prospects and existing customers are looking at on their website. And not in an anonymous form like the Google Analytics data. But they were broken down to every single contact that is in the database. Behaviour-based segmentation gives the company the opportunity, among other things, to achieve digitized 1: 1 communication with each customer based on interests (e.g. pages or products viewed) and thus to achieve more orders through the website.
Tip # 6: consider data protection
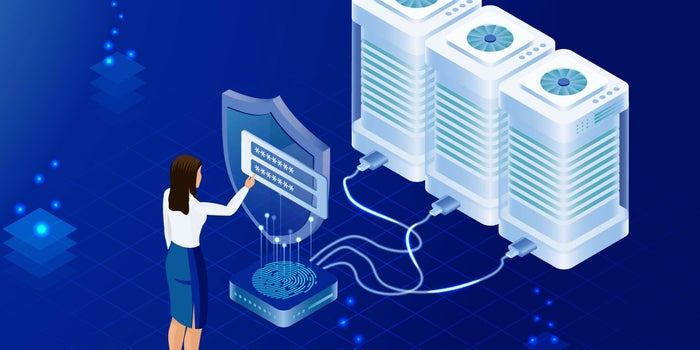
The valid data protection regulations must be complied with at every step of data management. Companies face a particular challenge here. On the one hand, the amount of data available in the company is increasing rapidly; on the other hand; it is often possible for many employees to access it. That is why companies have to pay more attention to personal and company data protection and security. In Switzerland, the provisions that are relevant to “offline” also apply to online marketing. This applies to contract law, the law against unfair competition, the price disclosure regulation, data protection law, copyright law and advertising law. If the trade focus is international, the foreign legal basis must also be taken into account. This is also important about the General Data Protection Regulation (GDPR).
Tip # 7: data enrichment
Data enrichment is the term used to describe the addition of “raw” data sets with additional information from a different source. The data enrichment very often combines external and internal data in a single profile. The applicable data protection regulations must also be observed when enriching data. Therefore, enhancing existing data sets is a relatively complex but very worthwhile task, as it leads to more prosperous, more up-to-date data sets. Today automatic systems can take over this task. Data enrichment tools use CRM, for example, to find additional, supplementary data. Enriching data is a process.
The following five use cases show why data enrichment is so significant:
- Fewer form fields required: A small number of form fields increases the probability of conversion significantly. Data enrichment tools add targeted B2B data in the background.
- A higher degree of personalization for every interaction: data enrichment creates a wealth of data that makes even tiny customer segments identifiable and specifically addressable. This enriched data allows automated interactions, for example, relating to recently held events in the account’s company.
- Advanced Account Scoring: Data enrichment is vital to automate lead and account scoring processes fully. Enriched data gives the scoring system even more information for making the right decisions.
- Improve the customer experience: Enriched CRM data makes it possible to tailor sales processes individually to interested parties, use upselling or cross-selling opportunities, and identify warning signals early. Real-time data enrichment helps companies to support their customers better.
- Use machine learning: AI applications, such as chatbots, collect critical data from website visitors. Enriched with further CRM data, a detailed view of the visitor’s wishes is created. Here, personalized touchpoints are made much more precisely and comprehensively automatically.
Tip # 8: data audit and employee training
A quarter to six-monthly data management audit should occur in the company so that the data quality can be consistently high in the long term. This illuminates the status of the data, its rate, the data flow and other factors. An additional check is carried out depending on the tools used, which ideally carry out an ongoing audit. In this context, the objectives of employee training should also include the communication of current legal provisions. But understanding data must also be an issue. Team and employees must be made aware of the topic of data and data management.
Tip # 9: automation
Existing systems can be used to automate data management tasks, provided they exist. Corrected and standardized customer data sets with high quality are then automatically fed to the corresponding processes. Data quality assurance tools also allow the automated checking of existing data for reliability and consistency. First of all, the customer profiles are automatically converted into a standardized form, which simplifies the enrichment through additional channels or data sources. Customer and product data and historical data can then be automatically assigned to the correct contact. What used to be associated with time-consuming list comparisons is now done fully automatically in a short time. Suppose the data quality check can be automated, and a permanent data audit is carried out. In that case, a clear strategy is still essential because even high-quality data sets are only useful if they are supported by a suitable method.
Tip # 10: Predictive Analytics (eng. Predictive analysis)
Data-driven approaches, such as predictive analytics, depending on professional data management. The demographic data that has already been adjusted is supplemented with behavioural data or other information from a product database. Further signals from complementary sources are added to this data. This could include information about the technologies used by contacts and accounts and others. For predictive analytics, a model is created from this data that also consists of the existing historical success metrics. Such a model reveals variables in its data that correlate with a successful completion. Once created, this model can now be used to support data-driven marketing decisions. Lead scoring and account targeting is ideal for this purpose. But also nurturing and personalization, as well as other areas, benefit significantly from predictive analytics.
Data management – essential work for more efficiency
The use of data and its handling has changed a lot in the last few years, so there is no time to stick to traditional ways of thinking. Good data management has the unbeatable advantage of allowing a much more comprehensive view of customers and processes. Incredibly granular strategy development is then possible on this basis. Technological developments in artificial intelligence ensure improved user experience on several levels because predictive analytics allow much more individual personalization than ever before. Data management should take a central position in the organization to contribute to more business success. As a rule, this requires the adaptation of previous processes to become more efficient.
Good data management is an essential requirement for marketing automation.
Reliable, pre-structured and optimally enriched data make marketing automation possible in the first place. Good data management begins according to the principle of “Think big, start small.” Smaller SMEs already benefit from replacing a newsletter system (or an Excel list) with a marketing automation system. For example, they can see what potential customers are looking at on the website (through behaviour-based tracking) and thus receive essential data to understand their customers better. Larger companies are likely to be interested in CDP and Account-Based Marketing.